Install Armbian Debian Server onto NanoPi NEO
Hi, all, I flied back and forth between ShenZhen China and Vancouver Canada in January 2018, and took a holiday break in California USA during Chinese Spring Festival in Febrary 2018. Now, I'm back to Vancouver and write this blog. Today, we are going to talk about how to flash the most recent Linux Kernel onto an NanoPi NEO. The board looks like (cited from NanoPi NEO ):
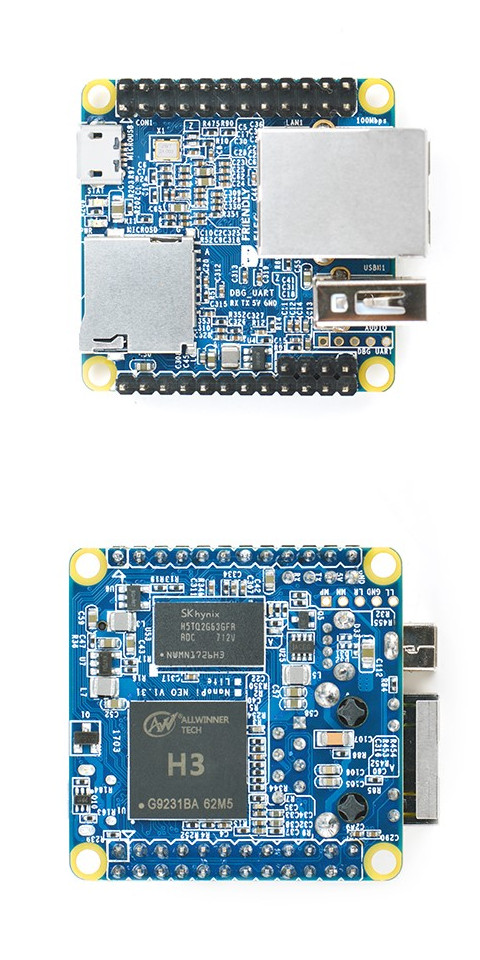
Different from our previous blog where U-Boot and Linux Kernel are manually built, here, we download and install the built Ubuntu from Armbian directly.
Before starting, the following paragraph is cited to explain the relationships among AllWinner, Sunxi and Linaro.
In march 2014, Allwinner joined Linaro as part of the new linaro digital home group. After this, Allwinner stopped communicating with the sunxi community, as linaro membership apparently satisfies the marketing need to be seen as an open source friendly company. Despite Linaro membership, Allwinner still violates the GPL on many counts. (cited from http://linux-sunxi.org/Allwinner .)
PART A: Install Debian Server Built By Armbian onto NanoPi NEO
1. Download Armbian Debian Server for NanoPi NEO
We FIRST go visiting the website https://www.armbian.com/nanopi-neo/ and click Debian server -- mainline kernel icon, a file named Armbian_5.38_Nanopineo_Debian_stretch_next_4.14.14.7z will be automatically downloaded.
Then, we extract this .7z file by
1 | $ 7za e Armbian_5.38_Nanopineo_Debian_stretch_next_4.14.14.7z |
2. Install Armbian Debian Server for NanoPi NEO
After the extracted image file is prepared, it's the time to install the Armbian Debian Server onto our TF card. We FIRST format the TF card:
1 | $ sudo mkfs.ext4 /dev/mmcblk0 |
Afterwards, use dd to install the downloaded Armbian Ubuntu Desktop image.
1 | $ sudo dd bs=4M if=Armbian_5.38_Nanopineo_Debian_stretch_next_4.14.14.img of=/dev/mmcblk0 conv=fsync |
PART B: Boot Into Armbian, Network Configuration and Armbian Upgrading
As known, NanoPi NEO comes with NEITHER a HDMI interface for display, NOR a Wifi interface for wireless network connection. Therefore, we may have to find a wired cable, and connect our NanoPi NEO to a router, which also connects with our host computer. Afterwards, we'll have to find our NanoPi NEO connection via router settings. Here in my case, I'm using a Cisco DPC3848V DOCSIS 3.0 Gateway, with the gateway IP: 192.168.0.1. By visiting http://192.168.0.1 -> Setup -> LAN Setup -> Connected Devices Summary, you should be able to find out which is the newly connected network device, namely NanoPi NEO.
1. SSH Into NanoPi NEO
Through our host computer, SSH into NanoPi NEO:
1 | $ ssh root@192.168.0.76 |
Then you input 1234 as the password, you'll be asked to change your password right away.
1 | You are required to change your password immediately (root enforced) |
And then you will be asked to create a new user account.
1 | Creating a new user account. Press <Ctrl-C> to abort |
2. Network Configuration
1) Doublecheck IP address
1 | root@nanopineo:~# ifconfig |
2) Modify /etc/network/interfaces
I noticed that when you reboot NanoPi NEO, the IP address changed from time to time. The reason why it is so is because the MAC address of NanoPi NEO change randomly after Armbian reboot. Therefore, it's recommended to manually set a static MAC address for NanoPi NEO in file /etc/network/interfaces.
1 | root@nanopineo:~# vim /etc/network/interfaces |
Just make sure to uncomment this line hwaddress ether # if you want to set MAC manually and write in a fixed MAC address. The final /etc/network/interfaces for my NanoPi NEO is as follows:
1 | root@nanopineo:~# cat /etc/network/interfaces |
After a while, ssh into Armbian with created user nanopineo:
1 | $ ssh nanopineo@192.168.0.36 |
4. Armbian Upgrading
Then we simply upgrade Armbian.
1 | nanopineo@nanopineo:~$ sudo apt update |
5. Kernel Doublechecking
Finally, we have the system and kernel doublechecked.
1 | nanopineo@nanopineo:~$ uname -r |